
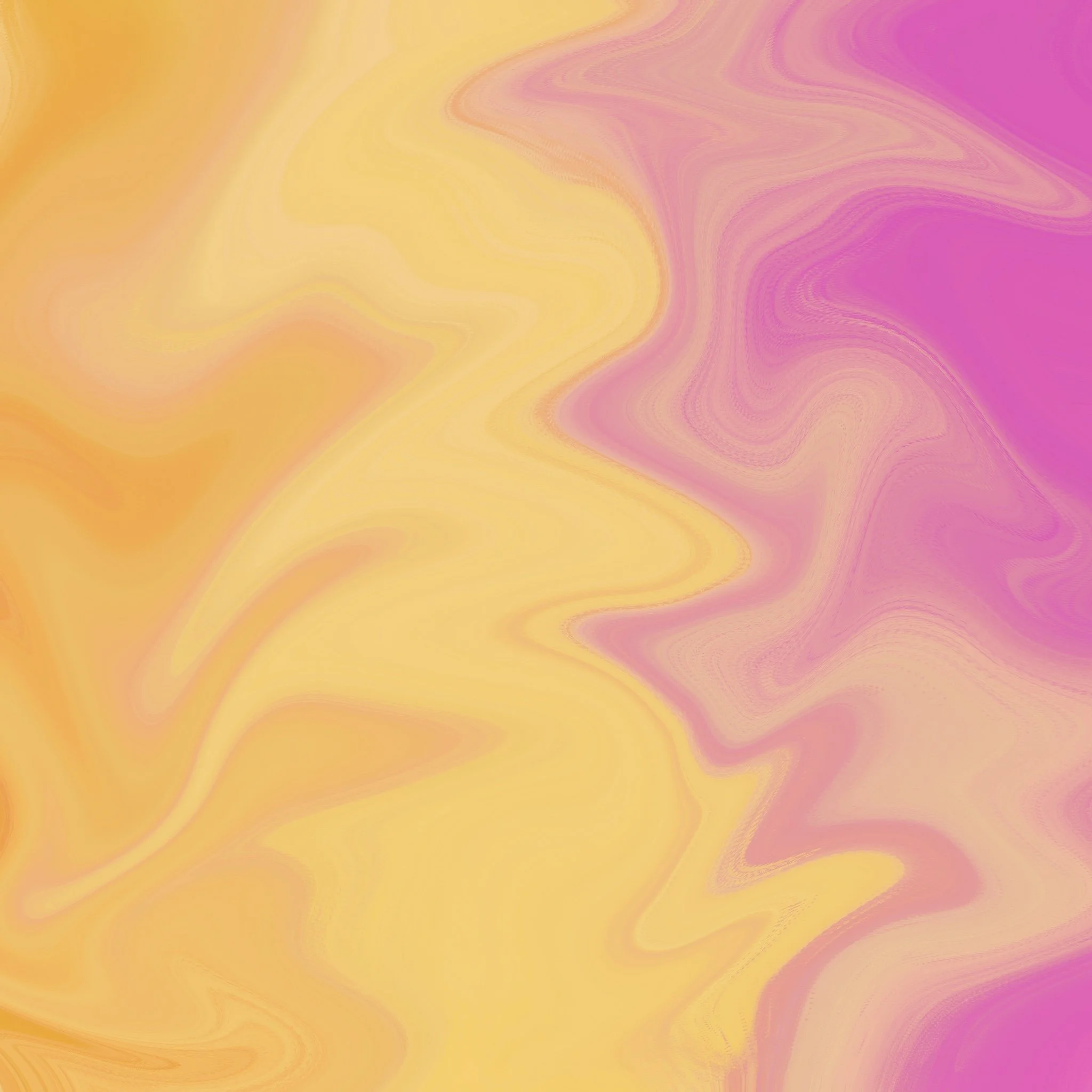
Everything You Need to Know About PINNs
Physics-Informed Neural Networks (PINNs) combine traditional AI with fundamental physics principles, enabling accurate predictions with minimal data requirements. Unlike conventional neural networks that require vast amounts of training data, PINNs incorporate physical laws directly into their learning process, making them highly efficient for solving complex scientific problems. These revolutionary networks are being successfully applied across various fields, including fluid dynamics, quantum mechanics, structural engineering, and climate science.
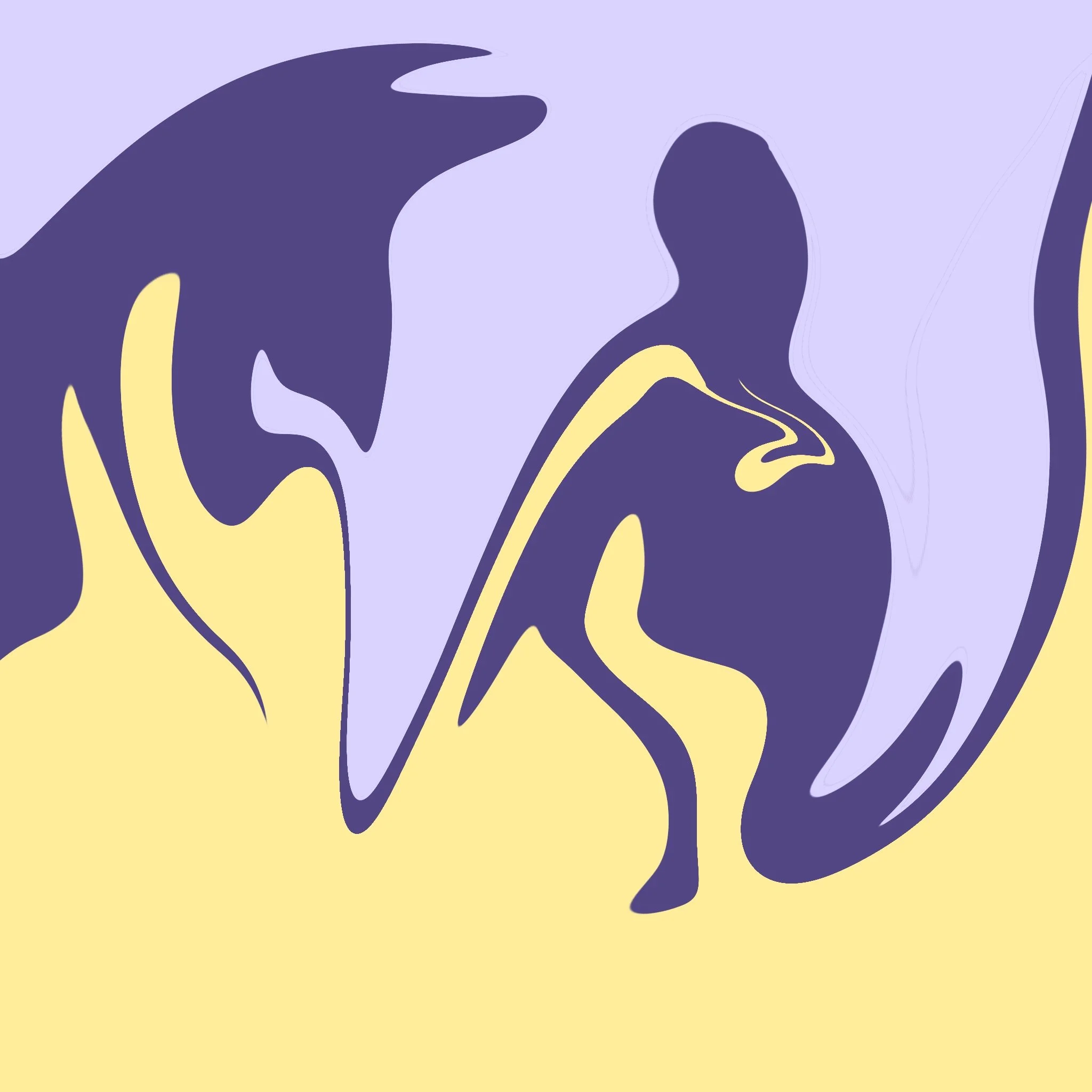
From Eruption to Innovation: Polydispersity’s Impact on Nature and Industry
This article explores groundbreaking research by Foster et al., which investigates the role of polydispersity in sedimenting flows like pyroclastic density currents (PDCs). By leveraging high-resolution simulations, the study highlights how particle diversity influences clustering, turbulence, and settling behavior, challenging traditional models. The findings offer a framework for more accurate predictions of natural phenomena and optimized designs in industrial and environmental systems.